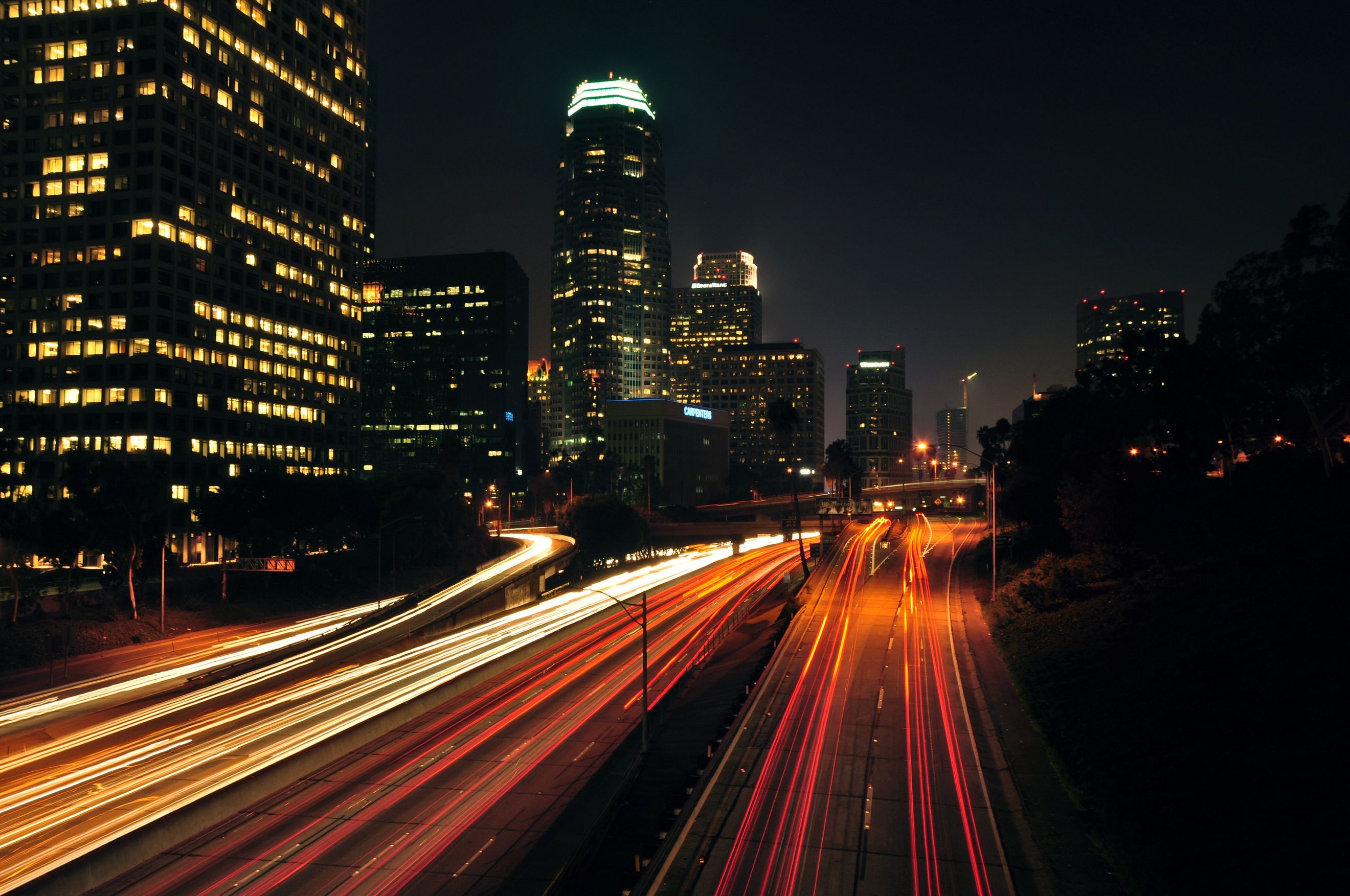
Smarter Cities Through AI-Powered Traffic Management: A Game Changer for Federal IT Services
Unleashing the Power of AI and ML to Transform Urban Mobility and Reduce Emissions
Taking Control of the Traffic Pandemonium
In a world where cities are becoming more congested and urban populations continue to grow, traffic management remains a significant challenge for municipal authorities and federal IT services alike. Traditional traffic management systems have fallen short in addressing the increasingly complex demands of urban mobility. However, recent advances in artificial intelligence (AI) and machine learning (ML) promise to revolutionize how we navigate our cities, improving transportation efficiency and reducing emissions.
This article explores the potential of smart traffic management systems that utilize AI and ML to analyze traffic patterns, optimize traffic signal timings, and predict congestion. It also provides valuable insights and actionable tips tailored to the unique needs and requirements of federal IT services, empowering them to implement cutting-edge solutions for the benefit of all.
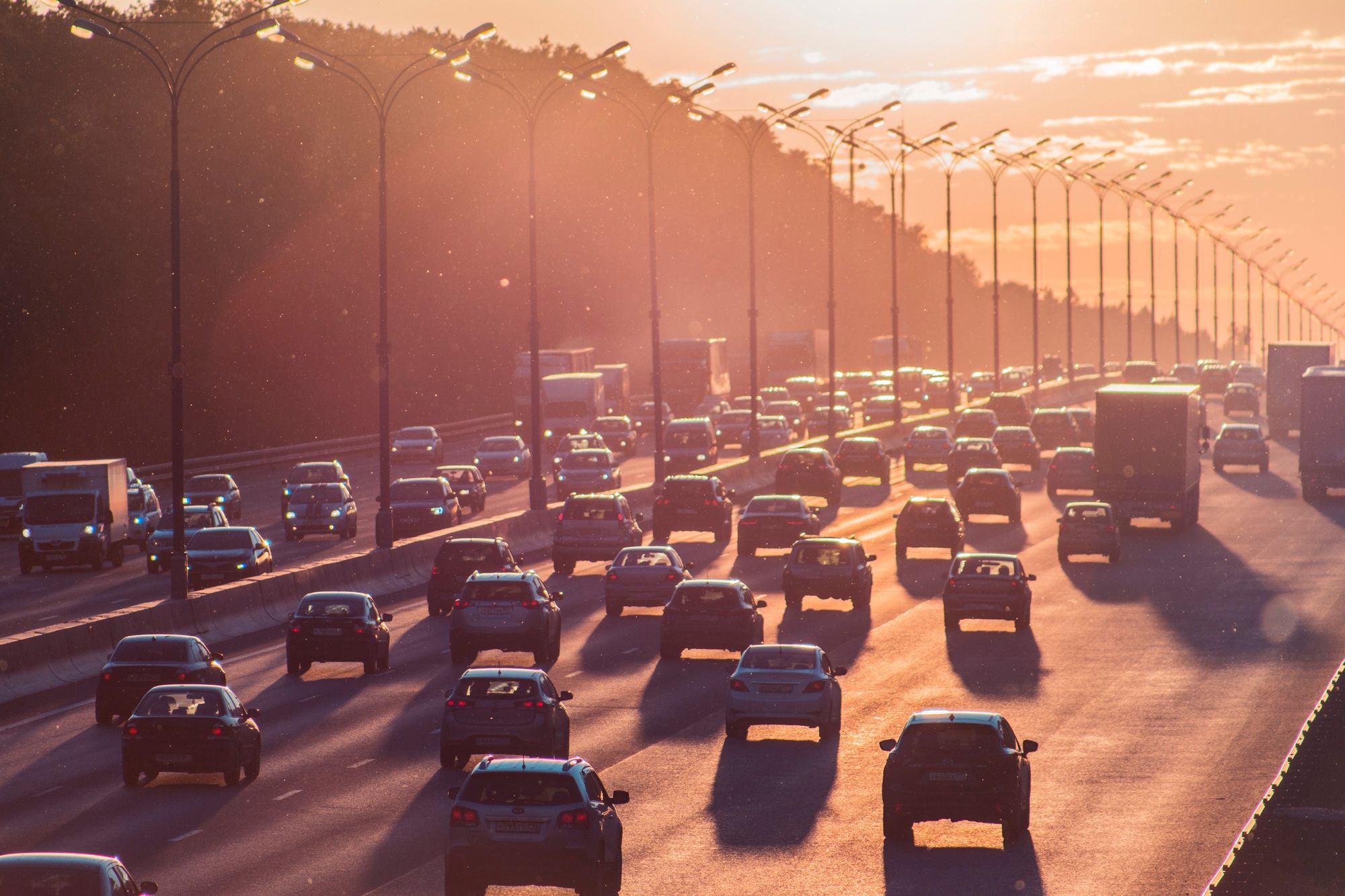
Unraveling the AI and ML Traffic Management Revolution
Analyzing Traffic Patterns with Unprecedented Precision
One of the critical components of an AI-powered traffic management system is its ability to analyze complex traffic patterns accurately. By leveraging data collected from various sources, such as cameras, sensors, and connected devices, AI algorithms can construct a real-time, comprehensive picture of traffic conditions. This information enables traffic controllers to make informed decisions and adapt signal timings, ultimately improving traffic flow and reducing congestion.
Actionable Tip: Federal IT services should invest in the development and implementation of advanced data collection and analysis tools, ensuring a steady flow of accurate and relevant traffic data for AI algorithms to work with.
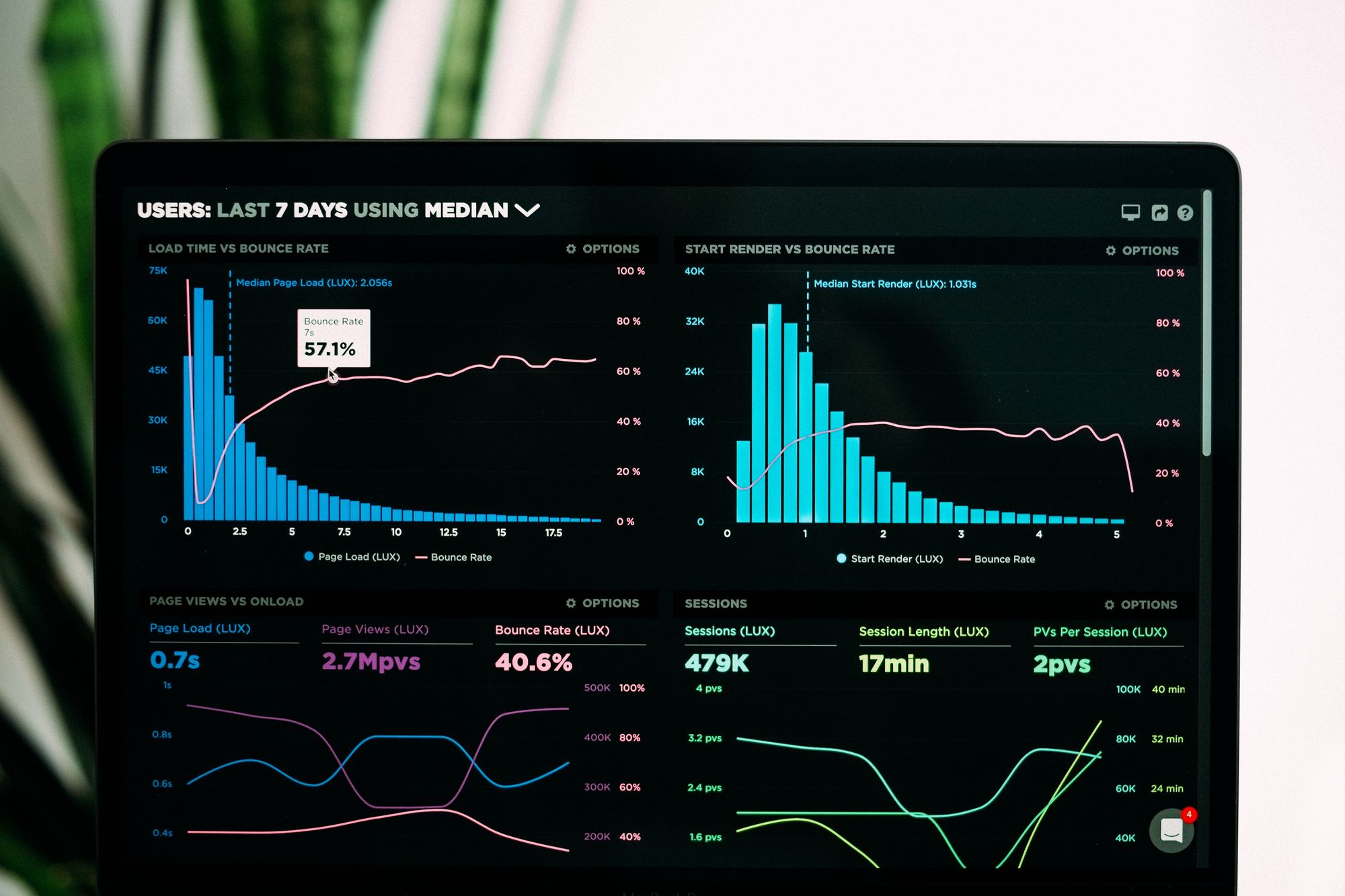
Optimizing Traffic Signal Timings for Maximum Efficiency
Traditional traffic signal timings often rely on fixed schedules or simplistic sensor-based systems that fail to account for the complex and dynamic nature of urban traffic. AI and ML can optimize traffic signal timings by continuously learning from traffic patterns and making predictions about future traffic conditions. This dynamic approach leads to better traffic flow, shorter waiting times at intersections, and a reduction in emissions from idling vehicles.
Actionable Tip: Federal IT staff should collaborate with local authorities and transportation experts to develop AI-driven adaptive traffic signal timing systems that can be scaled up and integrated into existing infrastructure.
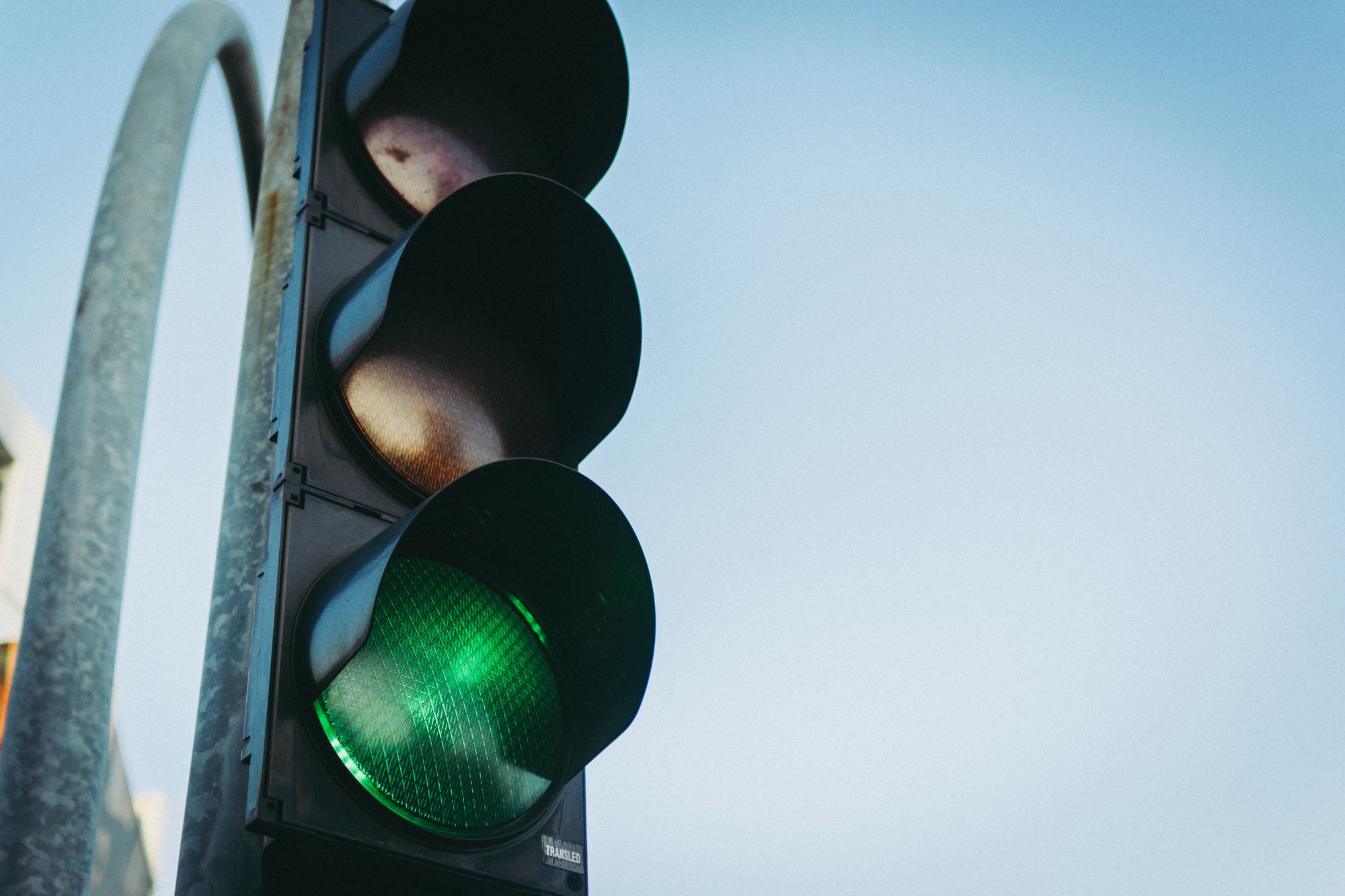
Predicting and Mitigating Congestion
AI and ML algorithms can go beyond merely reacting to traffic conditions. They can predict potential congestion and suggest proactive measures to avoid gridlock. By analyzing historical traffic data, weather forecasts, and information on special events, AI-driven traffic management systems can anticipate traffic bottlenecks and devise strategies to minimize their impact.
Actionable Tip: Federal IT services should engage in the development of predictive traffic models that account for various factors, such as seasonal variations and local events, to ensure accurate and reliable congestion forecasts.
Embracing the Future of Urban Mobility
Smart traffic management systems powered by AI and ML offer an unprecedented opportunity for federal IT services to lead the way in transforming urban mobility. By implementing advanced data analysis tools, optimizing traffic signal timings, and developing predictive traffic models, federal IT staff can make a significant impact on transportation efficiency and emission reduction. The time has come for cities to embrace this game-changing technology and create more sustainable, efficient, and livable urban environments for all.
Taking Control of the Traffic Pandemonium
In a world where cities are becoming more congested and urban populations continue to grow, traffic management remains a significant challenge for municipal authorities and federal IT services alike. Traditional traffic management systems have fallen short in addressing the increasingly complex demands of urban mobility. However, recent advances in artificial intelligence (AI) and machine learning (ML) promise to revolutionize how we navigate our cities, improving transportation efficiency and reducing emissions.
This article explores the potential of smart traffic management systems that utilize AI and ML to analyze traffic patterns, optimize traffic signal timings, and predict congestion. It also provides valuable insights and actionable tips tailored to the unique needs and requirements of federal IT services, empowering them to implement cutting-edge solutions for the benefit of all.
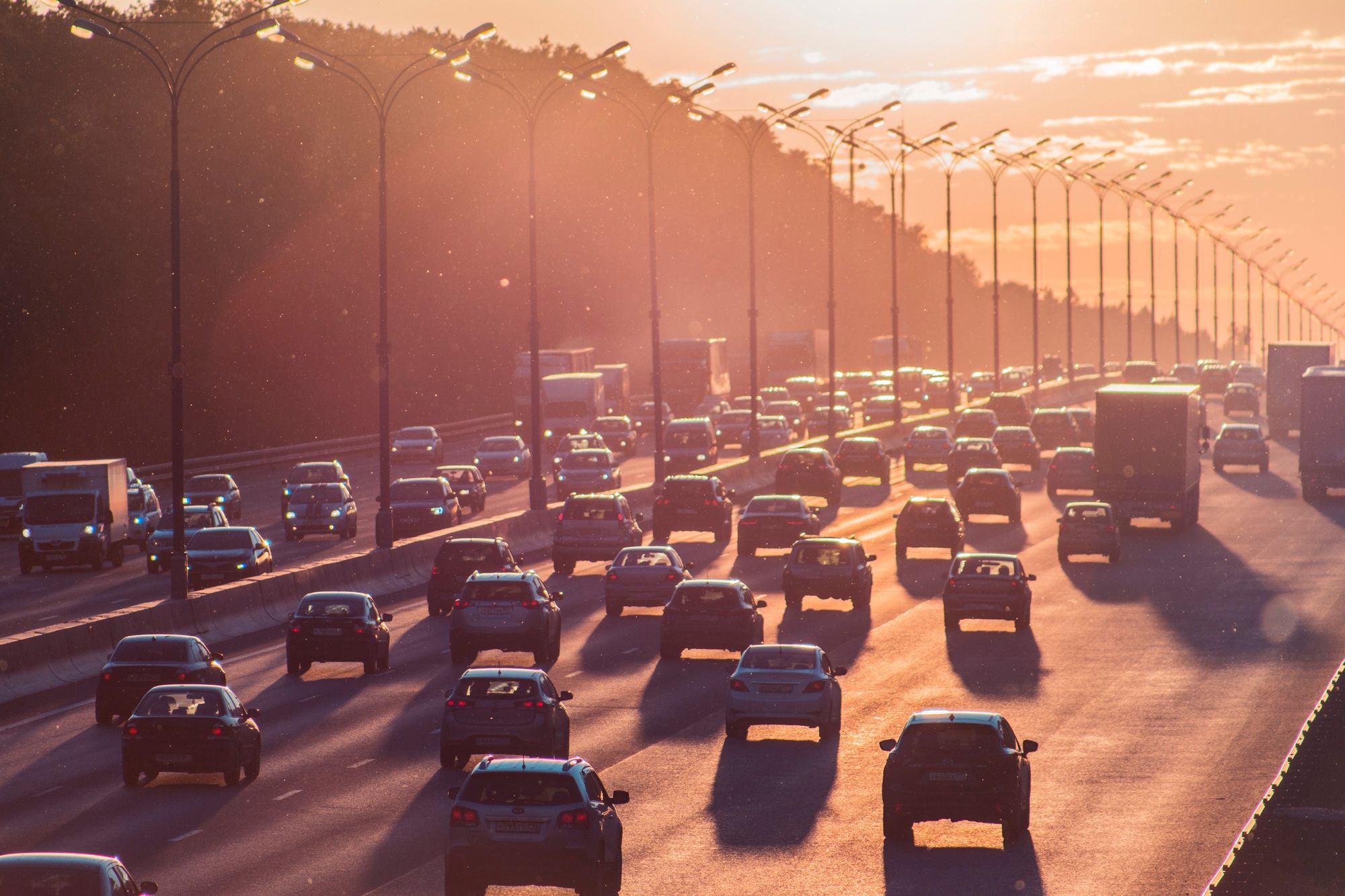
Unraveling the AI and ML Traffic Management Revolution
Analyzing Traffic Patterns with Unprecedented Precision
One of the critical components of an AI-powered traffic management system is its ability to analyze complex traffic patterns accurately. By leveraging data collected from various sources, such as cameras, sensors, and connected devices, AI algorithms can construct a real-time, comprehensive picture of traffic conditions. This information enables traffic controllers to make informed decisions and adapt signal timings, ultimately improving traffic flow and reducing congestion.
Actionable Tip: Federal IT services should invest in the development and implementation of advanced data collection and analysis tools, ensuring a steady flow of accurate and relevant traffic data for AI algorithms to work with.
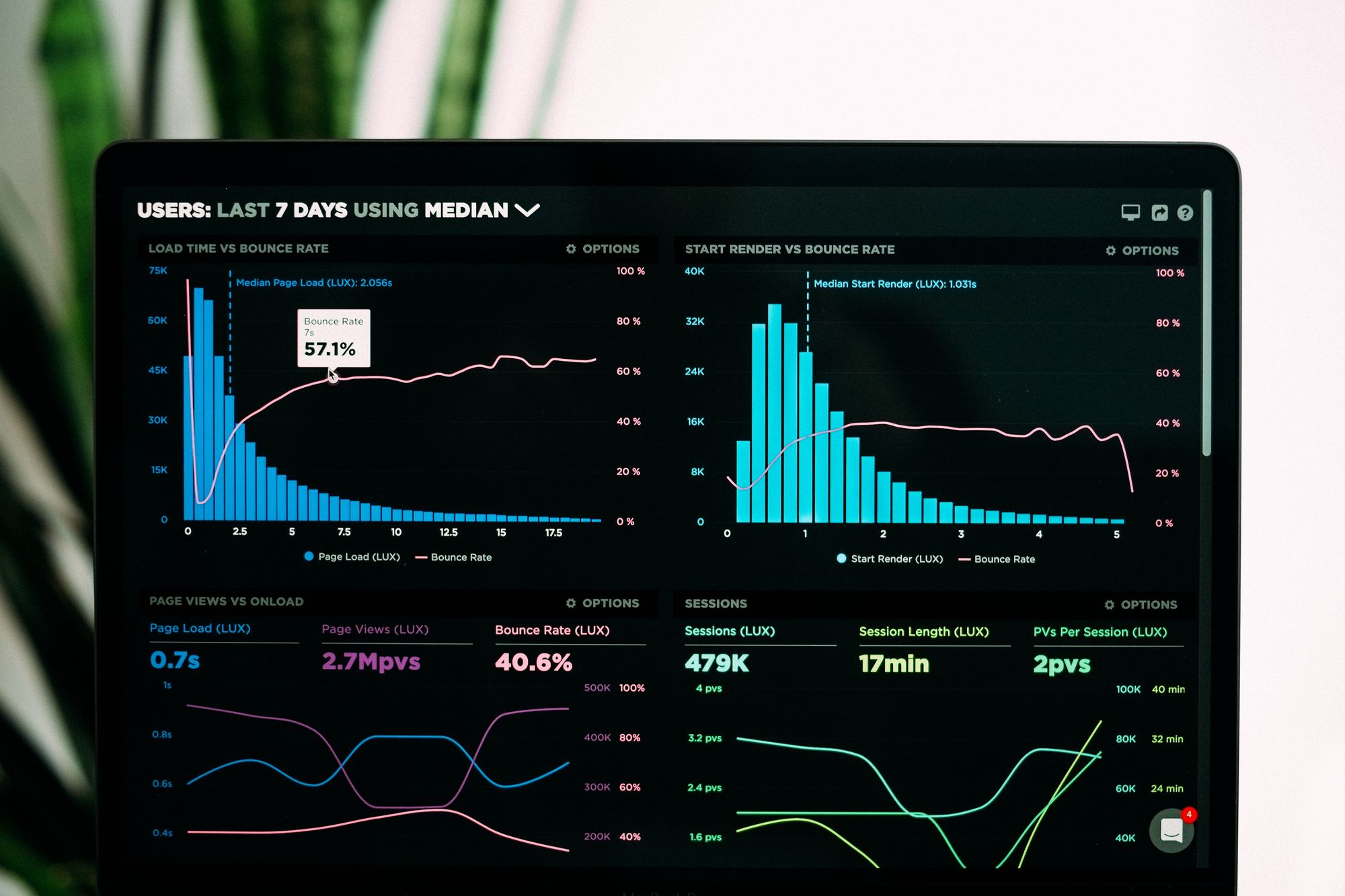
Optimizing Traffic Signal Timings for Maximum Efficiency
Traditional traffic signal timings often rely on fixed schedules or simplistic sensor-based systems that fail to account for the complex and dynamic nature of urban traffic. AI and ML can optimize traffic signal timings by continuously learning from traffic patterns and making predictions about future traffic conditions. This dynamic approach leads to better traffic flow, shorter waiting times at intersections, and a reduction in emissions from idling vehicles.
Actionable Tip: Federal IT staff should collaborate with local authorities and transportation experts to develop AI-driven adaptive traffic signal timing systems that can be scaled up and integrated into existing infrastructure.
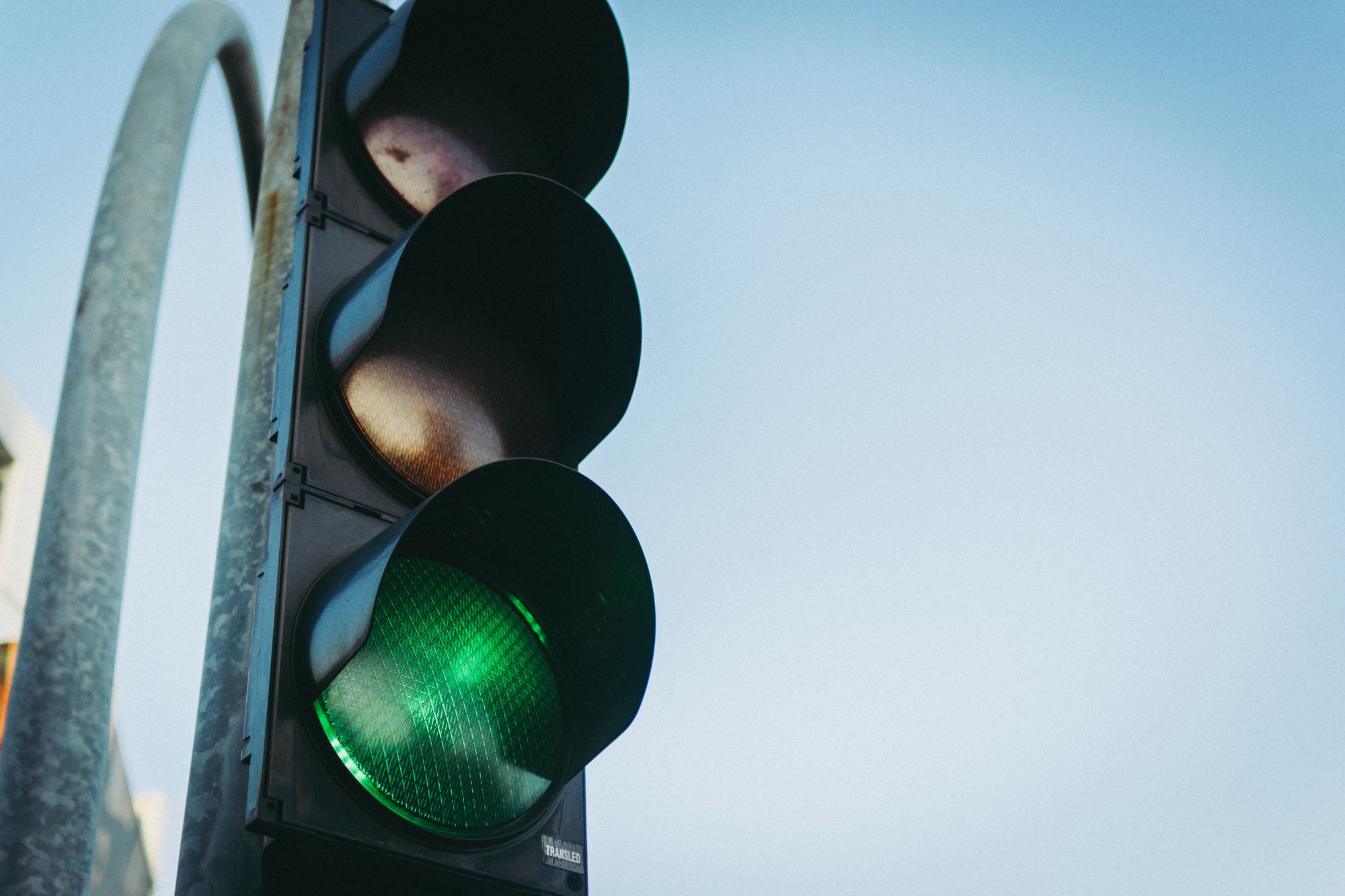
Predicting and Mitigating Congestion
AI and ML algorithms can go beyond merely reacting to traffic conditions. They can predict potential congestion and suggest proactive measures to avoid gridlock. By analyzing historical traffic data, weather forecasts, and information on special events, AI-driven traffic management systems can anticipate traffic bottlenecks and devise strategies to minimize their impact.
Actionable Tip: Federal IT services should engage in the development of predictive traffic models that account for various factors, such as seasonal variations and local events, to ensure accurate and reliable congestion forecasts.
Embracing the Future of Urban Mobility
Smart traffic management systems powered by AI and ML offer an unprecedented opportunity for federal IT services to lead the way in transforming urban mobility. By implementing advanced data analysis tools, optimizing traffic signal timings, and developing predictive traffic models, federal IT staff can make a significant impact on transportation efficiency and emission reduction. The time has come for cities to embrace this game-changing technology and create more sustainable, efficient, and livable urban environments for all.
'