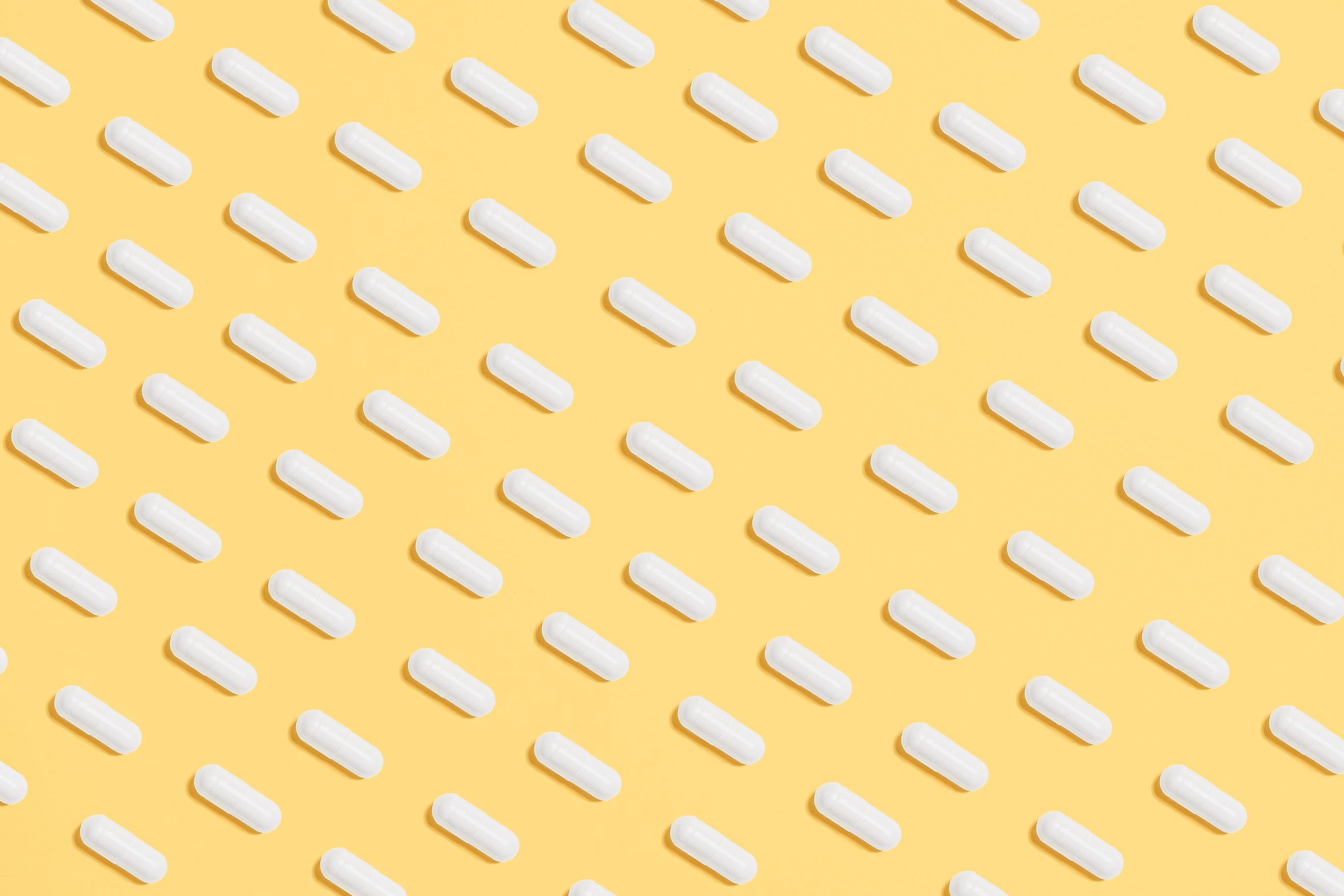
AI and Machine Learning in Drug Discovery and Development
As technology continues to advance, we can expect AI and machine learning to play an increasingly important role in shaping the future of drug discovery and development.
AI and machine learning have the potential to revolutionize drug discovery and development in several ways. These include identifying potential drug targets more efficiently, analyzing large libraries of compounds to identify promising molecules for further development, predicting potential adverse drug reactions, identifying patient subgroups that may respond differently to certain drugs, and enabling more personalized medicine. By enabling more efficient and accurate decision-making, improving drug safety and efficacy, and reducing the time and cost required for drug development, AI and machine learning can play a critical role in improving human health and addressing unmet medical needs.
AI, or artificial intelligence, refers to the ability of machines to mimic intelligent human behavior such as learning, reasoning, and problem-solving. Machine Learning (ML) is a subset of AI that involves training machines on large datasets to identify patterns and make predictions or decisions without being explicitly programmed. Essentially, machine learning algorithms learn from data and use that knowledge to make decisions or predictions.
AI and Machine Learning have had a significant impact on multiple industries, including:
Healthcare: AI and machine learning have enabled more accurate diagnoses and treatment plans, better disease detection and monitoring, and improved patient outcomes. For example, ML algorithms can analyze medical images to detect tumors, predict disease progression, and identify potential treatment options.
Finance: AI and machine learning have revolutionized the financial industry by enabling fraud detection, risk management, and automated trading. For example, ML algorithms can analyze large amounts of financial data to identify patterns that could indicate fraudulent activity.
Retail: AI and machine learning have enabled more personalized and efficient customer experiences through product recommendations and optimized supply chain management. For example, ML algorithms can analyze customer data to predict future buying behavior and make personalized product recommendations.
Manufacturing: AI and machine learning have enabled increased efficiency and productivity through predictive maintenance, supply chain optimization, and quality control. For example, ML algorithms can analyze sensor data to predict when equipment will need maintenance, reducing downtime and improving productivity.
Transportation: AI and machine learning have enabled self-driving cars and improved traffic management through predictive modelling and real-time data analysis. For example, ML algorithms can analyze traffic patterns to optimize routes and reduce congestion.
Overall, AI and Machine Learning have transformed multiple industries by enabling more efficient and accurate decision-making, improving customer experiences, and increasing productivity and profitability. As technology continues to advance, we can expect AI and Machine Learning to continue to play a significant role in shaping the future of many industries.
“Despite the challenges, drug discovery and development are essential for improving human health and addressing unmet medical needs.”
Drug discovery and development is a complex process that involves identifying and developing new medications or therapies to treat various diseases or medical conditions. The process typically involves several stages that require collaboration among researchers from various disciplines.
The first stage of drug discovery is target identification, which involves identifying the biological target or molecule that the drug will interact with to produce a therapeutic effect. Once the target has been identified, researchers move on to hit identification, which involves identifying and screening large libraries of compounds to find molecules that have the potential to interact with the target and produce a therapeutic effect.
Once a promising molecule has been identified, researchers move on to lead optimization, where they optimize its properties to improve its efficacy, safety, and other desirable characteristics. This is a critical stage in drug discovery, as the properties of the lead molecule will determine its suitability for further development.
Before a drug can be tested in humans, it must undergo rigorous testing in animals to evaluate its safety and effectiveness. This stage is known as preclinical testing and is essential for ensuring that the drug is safe and effective before it is tested in humans.
If a drug shows promising results in preclinical testing, it will move on to clinical trials, which involve testing the drug in humans to evaluate its safety, efficacy, and optimal dosing. Clinical trials are typically conducted in several phases and involve progressively larger groups of human subjects.
If a drug successfully completes clinical trials and is found to be safe and effective, it can be submitted for regulatory approval by government agencies such as the FDA in the United States. The regulatory approval process is a rigorous and lengthy process that requires extensive documentation of the drug's safety, efficacy, and manufacturing processes.
Despite the challenges, drug discovery and development are essential for improving human health and addressing unmet medical needs. The process requires the collaboration of researchers from various disciplines, including chemistry, biology, pharmacology, and medicine, and can take years or even decades to complete. However, the potential benefits of new medications or therapies make the effort worthwhile.
“As technology continues to advance, we can expect AI and machine learning to play an increasingly important role in shaping the future of drug discovery and development.”
AI and machine learning have the potential to transform drug discovery and development by enabling more efficient and accurate decision-making, improving drug safety and efficacy, and reducing the time and cost required for drug development.
One of the most promising applications of AI and machine learning in drug discovery is target identification. AI algorithms can analyze large datasets to identify potential targets for drug development, including new disease pathways or biological mechanisms. This can significantly reduce the time and cost required for target identification, enabling researchers to focus their efforts on the most promising targets.
Another promising application of AI and machine learning is in the early stages of drug development, including hit identification and lead optimization. AI algorithms can analyze large libraries of compounds to identify molecules with the most promising therapeutic potential. This can significantly accelerate the drug development process by reducing the time and resources required for hit identification and lead optimization.
In addition to accelerating drug development, AI and machine learning can also improve drug safety and efficacy. For example, machine learning algorithms can analyze large datasets to predict potential adverse drug reactions and identify patients who may be at higher risk for adverse events. This can help researchers identify and address potential safety concerns earlier in the drug development process, reducing the likelihood of adverse events in clinical trials and post-market surveillance.
Furthermore, AI and machine learning can enable more personalized medicine by analyzing large datasets to identify patient subgroups that may respond differently to certain drugs. This can help researchers identify patients who are most likely to benefit from a particular drug, reducing the time and cost required for clinical trials and improving patient outcomes.
Overall, AI and machine learning have the potential to transform drug discovery and development by enabling more efficient and accurate decision-making, improving drug safety and efficacy, and reducing the time and cost required for drug development. As technology continues to advance, we can expect AI and machine learning to play an increasingly important role in shaping the future of drug discovery and development.
AI and machine learning have the potential to revolutionize drug discovery and development in several ways. These include identifying potential drug targets more efficiently, analyzing large libraries of compounds to identify promising molecules for further development, predicting potential adverse drug reactions, identifying patient subgroups that may respond differently to certain drugs, and enabling more personalized medicine. By enabling more efficient and accurate decision-making, improving drug safety and efficacy, and reducing the time and cost required for drug development, AI and machine learning can play a critical role in improving human health and addressing unmet medical needs.
AI, or artificial intelligence, refers to the ability of machines to mimic intelligent human behavior such as learning, reasoning, and problem-solving. Machine Learning (ML) is a subset of AI that involves training machines on large datasets to identify patterns and make predictions or decisions without being explicitly programmed. Essentially, machine learning algorithms learn from data and use that knowledge to make decisions or predictions.
AI and Machine Learning have had a significant impact on multiple industries, including:
Healthcare: AI and machine learning have enabled more accurate diagnoses and treatment plans, better disease detection and monitoring, and improved patient outcomes. For example, ML algorithms can analyze medical images to detect tumors, predict disease progression, and identify potential treatment options.
Finance: AI and machine learning have revolutionized the financial industry by enabling fraud detection, risk management, and automated trading. For example, ML algorithms can analyze large amounts of financial data to identify patterns that could indicate fraudulent activity.
Retail: AI and machine learning have enabled more personalized and efficient customer experiences through product recommendations and optimized supply chain management. For example, ML algorithms can analyze customer data to predict future buying behavior and make personalized product recommendations.
Manufacturing: AI and machine learning have enabled increased efficiency and productivity through predictive maintenance, supply chain optimization, and quality control. For example, ML algorithms can analyze sensor data to predict when equipment will need maintenance, reducing downtime and improving productivity.
Transportation: AI and machine learning have enabled self-driving cars and improved traffic management through predictive modelling and real-time data analysis. For example, ML algorithms can analyze traffic patterns to optimize routes and reduce congestion.
Overall, AI and Machine Learning have transformed multiple industries by enabling more efficient and accurate decision-making, improving customer experiences, and increasing productivity and profitability. As technology continues to advance, we can expect AI and Machine Learning to continue to play a significant role in shaping the future of many industries.
“Despite the challenges, drug discovery and development are essential for improving human health and addressing unmet medical needs.”
Drug discovery and development is a complex process that involves identifying and developing new medications or therapies to treat various diseases or medical conditions. The process typically involves several stages that require collaboration among researchers from various disciplines.
The first stage of drug discovery is target identification, which involves identifying the biological target or molecule that the drug will interact with to produce a therapeutic effect. Once the target has been identified, researchers move on to hit identification, which involves identifying and screening large libraries of compounds to find molecules that have the potential to interact with the target and produce a therapeutic effect.
Once a promising molecule has been identified, researchers move on to lead optimization, where they optimize its properties to improve its efficacy, safety, and other desirable characteristics. This is a critical stage in drug discovery, as the properties of the lead molecule will determine its suitability for further development.
Before a drug can be tested in humans, it must undergo rigorous testing in animals to evaluate its safety and effectiveness. This stage is known as preclinical testing and is essential for ensuring that the drug is safe and effective before it is tested in humans.
If a drug shows promising results in preclinical testing, it will move on to clinical trials, which involve testing the drug in humans to evaluate its safety, efficacy, and optimal dosing. Clinical trials are typically conducted in several phases and involve progressively larger groups of human subjects.
If a drug successfully completes clinical trials and is found to be safe and effective, it can be submitted for regulatory approval by government agencies such as the FDA in the United States. The regulatory approval process is a rigorous and lengthy process that requires extensive documentation of the drug's safety, efficacy, and manufacturing processes.
Despite the challenges, drug discovery and development are essential for improving human health and addressing unmet medical needs. The process requires the collaboration of researchers from various disciplines, including chemistry, biology, pharmacology, and medicine, and can take years or even decades to complete. However, the potential benefits of new medications or therapies make the effort worthwhile.
“As technology continues to advance, we can expect AI and machine learning to play an increasingly important role in shaping the future of drug discovery and development.”
AI and machine learning have the potential to transform drug discovery and development by enabling more efficient and accurate decision-making, improving drug safety and efficacy, and reducing the time and cost required for drug development.
One of the most promising applications of AI and machine learning in drug discovery is target identification. AI algorithms can analyze large datasets to identify potential targets for drug development, including new disease pathways or biological mechanisms. This can significantly reduce the time and cost required for target identification, enabling researchers to focus their efforts on the most promising targets.
Another promising application of AI and machine learning is in the early stages of drug development, including hit identification and lead optimization. AI algorithms can analyze large libraries of compounds to identify molecules with the most promising therapeutic potential. This can significantly accelerate the drug development process by reducing the time and resources required for hit identification and lead optimization.
In addition to accelerating drug development, AI and machine learning can also improve drug safety and efficacy. For example, machine learning algorithms can analyze large datasets to predict potential adverse drug reactions and identify patients who may be at higher risk for adverse events. This can help researchers identify and address potential safety concerns earlier in the drug development process, reducing the likelihood of adverse events in clinical trials and post-market surveillance.
Furthermore, AI and machine learning can enable more personalized medicine by analyzing large datasets to identify patient subgroups that may respond differently to certain drugs. This can help researchers identify patients who are most likely to benefit from a particular drug, reducing the time and cost required for clinical trials and improving patient outcomes.
Overall, AI and machine learning have the potential to transform drug discovery and development by enabling more efficient and accurate decision-making, improving drug safety and efficacy, and reducing the time and cost required for drug development. As technology continues to advance, we can expect AI and machine learning to play an increasingly important role in shaping the future of drug discovery and development.
'